The Rise of Autonomous AI Agents: A Comprehensive Guide to Their Architecture, Applications, and Impact
Dive into the architecture of autonomous AI agents, examining how Profile, Memory, Planning, and Action components work in harmony to create intelligent systems. Discover how these core elements enable agents to learn, adapt, and execute complex tasks while maintaining purposeful behavior
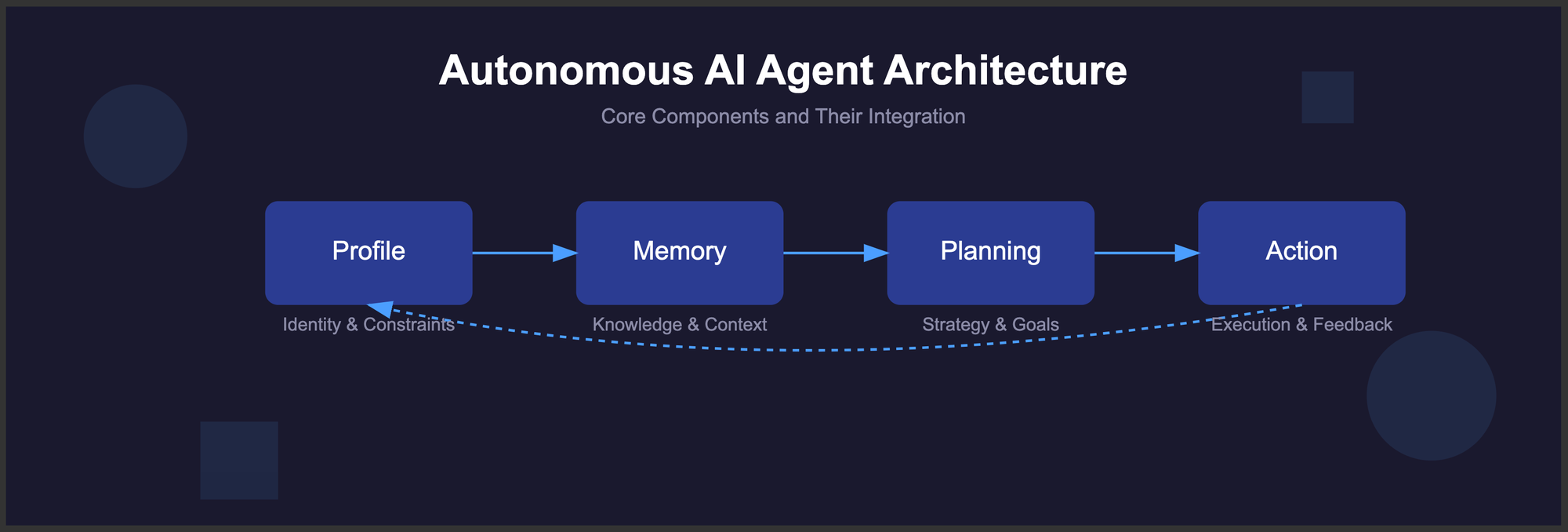
As we venture deeper into the age of artificial intelligence, autonomous AI agents have emerged as one of the most fascinating and transformative technologies of our time. These sophisticated systems, capable of independent operation and decision-making, are reshaping industries and challenging our understanding of machine capabilities. In this comprehensive exploration, we'll delve into the intricate world of autonomous AI agents, examining their architecture, applications, and the profound impact they're having on our world.
Understanding Autonomous AI Agents: A Deep Dive
The Evolution of AI Agents
The journey of autonomous AI agents begins with understanding their evolutionary path. Unlike traditional software systems that operate within rigid, predetermined parameters, autonomous AI agents represent a quantum leap in computational capability. These systems embody the culmination of decades of research in artificial intelligence, combining advances in machine learning, natural language processing, computer vision, and cognitive computing.
What sets autonomous AI agents apart is their ability to operate with minimal human intervention while adapting to new situations and learning from experience. This marks a significant departure from conventional automation tools that require explicit programming for every possible scenario they might encounter.
Defining Characteristics of Autonomous AI Agents
At their core, autonomous AI agents possess several defining characteristics that distinguish them from other AI systems:
- Autonomy: The ability to operate independently and make decisions without constant human oversight.
- Reactivity: Quick response capabilities to changes in their environment.
- Proactivity: The capacity to take initiative and pursue goals.
- Social Ability: The capability to interact with other agents or humans.
- Learning Capacity: The ability to improve performance through experience.
The Architecture of Autonomous AI Agents: The Four Core Components
At the foundation of every autonomous AI agent lies a sophisticated architecture built upon four essential components: Profile, Memory, Planning, and Action. These interconnected systems work in harmony to create an intelligent entity capable of understanding its purpose, learning from experience, formulating strategies, and executing actions effectively. Let's explore each core component in detail to understand how they contribute to the agent's overall capabilities.
The Profile Component: Defining Identity and Purpose
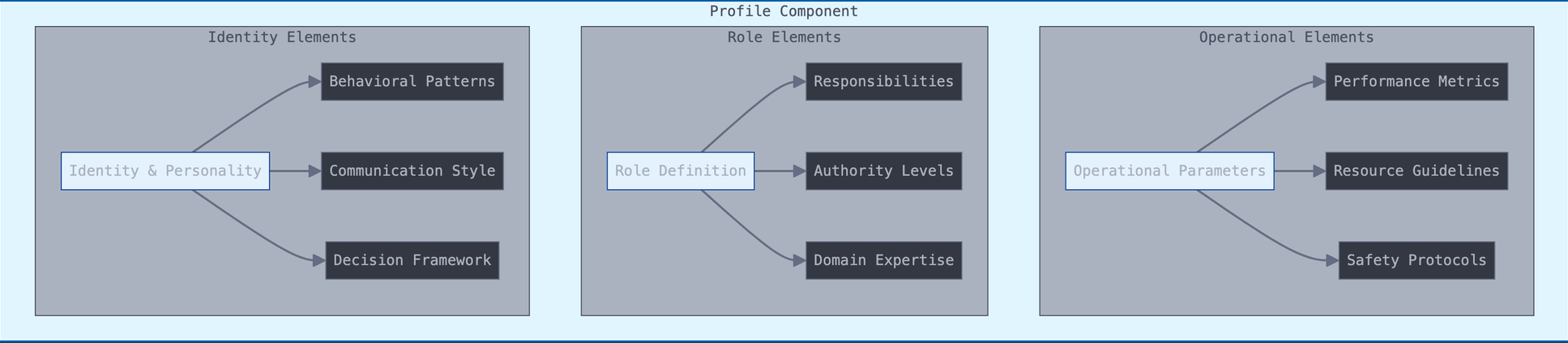
The Profile component serves as the agent's fundamental identity framework, encompassing several crucial elements:
Identity and Personality
The agent's profile establishes its core characteristics, including:
- Behavioral tendencies and interaction styles
- Communication preferences and patterns
- Decision-making approaches
- Ethical frameworks and constraints
- Response patterns to different situations
Role Definition
The profile clearly defines:
- Primary and secondary functional roles
- Scope of responsibilities
- Authority levels and limitations
- Interaction protocols with humans and other agents
- Domain-specific expertise areas
Operational Parameters
These include:
- Performance metrics and success criteria
- Resource utilization guidelines
- Priority frameworks for task execution
- Compliance requirements and regulatory constraints
- Safety protocols and operational boundaries
The Memory Component: Building Experience and Knowledge

The Memory component functions as the agent's cognitive foundation, incorporating sophisticated systems for information storage and retrieval:
Short-term Memory
This component manages:
- Current context and active tasks
- Recent interactions and their outcomes
- Temporary data needed for immediate operations
- Working memory for complex calculations
- Immediate environmental feedback
Long-term Memory
The long-term memory system handles:
- Historical interaction patterns
- Learned behaviors and successful strategies
- Domain knowledge and expertise
- Past experiences and their outcomes
- Performance optimization patterns
Memory Integration
The integration system ensures:
- Seamless transition between short and long-term memory
- Pattern recognition across experiences
- Knowledge consolidation and organization
- Efficient information retrieval
- Continuous learning and adaptation
The Planning Component: Strategic Thinking and Decision Making
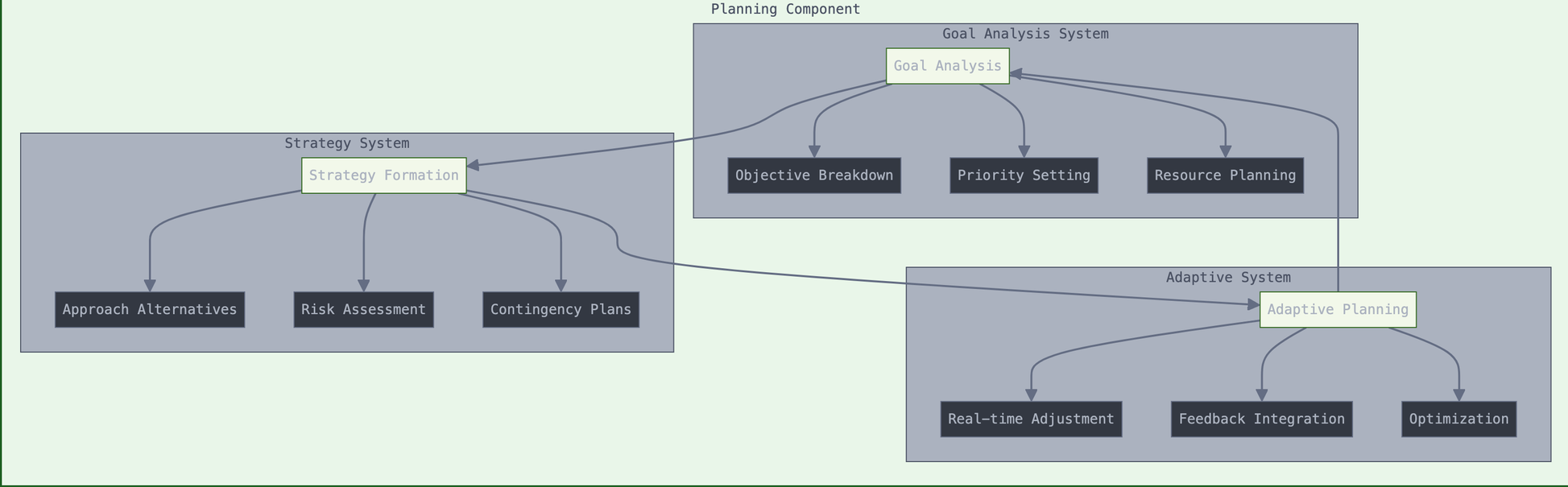
The Planning component enables the agent to formulate effective strategies and make informed decisions:
Goal Analysis
This involves:
- Breaking down complex objectives
- Identifying dependencies and prerequisites
- Prioritizing subtasks
- Resource allocation planning
- Timeline development
Strategy Formation
The planning system develops:
- Multiple approach alternatives
- Risk assessment frameworks
- Contingency plans
- Resource optimization strategies
- Performance monitoring methods
Adaptive Planning
This capability ensures:
- Real-time plan adjustment
- Response to unexpected events
- Learning from plan execution
- Optimization of future planning
- Integration of new constraints or opportunities
The Action Component: Execution and Implementation
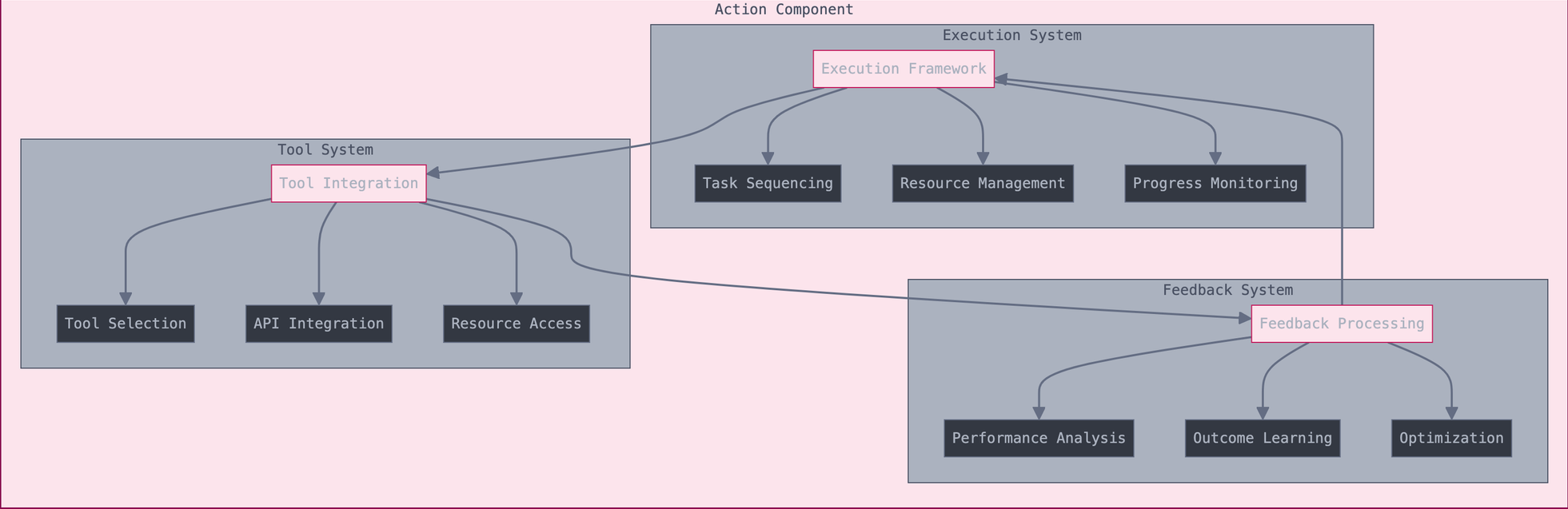
The Action component transforms plans into tangible outcomes through sophisticated execution mechanisms:
Execution Framework
This includes:
- Task sequencing and coordination
- Resource management
- Progress monitoring
- Error handling
- Performance optimization
Tool Integration
The action system manages:
- External tool selection and utilization
- API interactions
- Resource access and control
- Output formatting and delivery
- System integration
Feedback Processing
This component handles:
- Real-time performance monitoring
- Success/failure analysis
- Adjustment of execution parameters
- Learning from outcomes
- Optimization of future actions
Integration of the Four Core Components
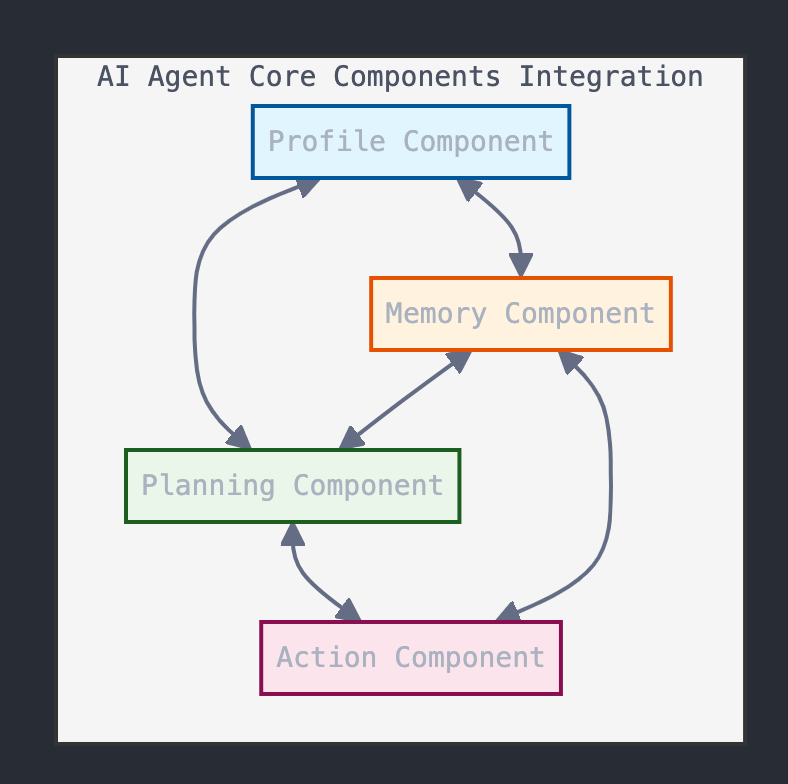
The true power of autonomous AI agents emerges from the seamless integration of these four core components. This integration enables:
The architecture of an autonomous AI agent consists of several sophisticated components working in harmony. Let's examine each component in detail:
1. Perception System
The perception system serves as the agent's sensory interface with the world. This component processes various types of input:
- Visual data through computer vision algorithms
- Audio information through speech recognition systems
- Textual data through natural language processing
- Sensor data from various environmental monitors
- Network and system metrics in digital environments
The perception system must not only gather this data but also preprocess it into a format that the agent's reasoning system can effectively utilize.
2. Knowledge Base
The knowledge base represents the agent's understanding of its domain and includes:
- Domain-specific knowledge and rules
- Historical data and learned patterns
- Operational constraints and parameters
- Goal hierarchies and success criteria
- Environmental models and predictions
This component acts as both a repository of information and a framework for understanding new information in context.
3. Reasoning Engine
The reasoning engine is perhaps the most complex component, responsible for:
- Analyzing perceived information against stored knowledge
- Identifying patterns and relationships in data
- Evaluating potential actions and their consequences
- Managing uncertainty and incomplete information
- Maintaining internal state consistency
Modern reasoning engines often employ a combination of approaches:
- Rule-based reasoning for well-defined scenarios
- Probabilistic reasoning for handling uncertainty
- Case-based reasoning for learning from past experiences
- Neural networks for pattern recognition and prediction
4. Decision-Making Module
The decision-making module transforms reasoning outputs into actionable decisions. This component:
- Evaluates multiple possible courses of action
- Considers resource constraints and limitations
- Balances short-term and long-term objectives
- Implements decision policies and strategies
- Manages risk and uncertainty in decision-making
5. Action Execution System
The action execution system translates decisions into concrete actions:
- Coordinates multiple actuators or system components
- Monitors action progress and completion
- Handles error conditions and unexpected situations
- Provides feedback to the reasoning system
- Maintains operational safety constraints
Synergistic Operations
The integration of the four components creates a dynamic system where:
- The Profile guides the Planning process by providing constraints and priorities
- Memory informs both Planning and Action by providing historical context and learned patterns
- Planning directs Action while incorporating feedback from previous executions
- Action results update Memory and inform future Planning
- The entire system continuously evolves while maintaining consistency with the Profile
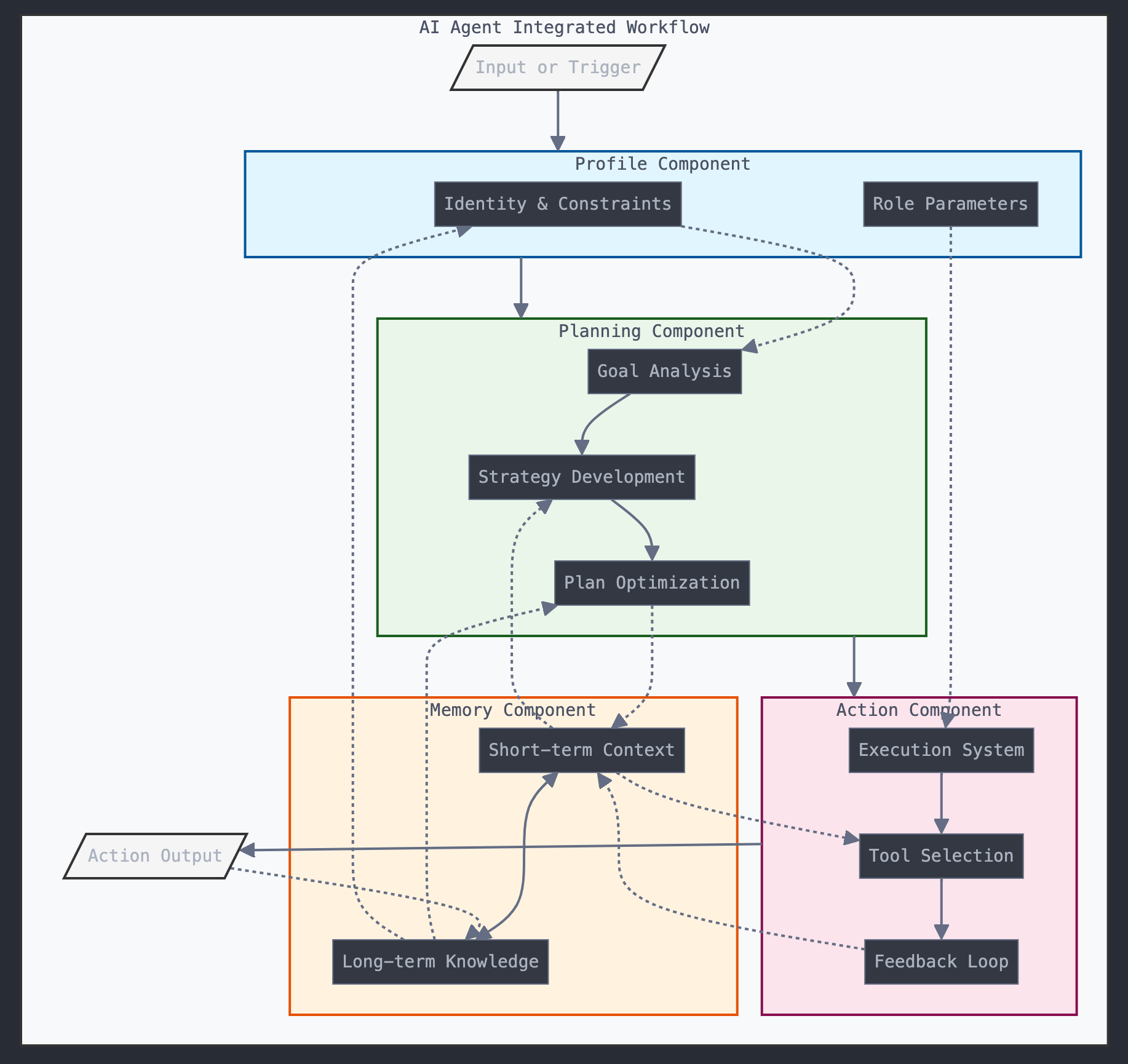
This interconnected architecture enables autonomous AI agents to:
- Learn and adapt while maintaining their core identity
- Make informed decisions based on accumulated experience
- Execute complex tasks with increasing efficiency
- Maintain consistency across various operations
- Build upon past successes while learning from failures
Applications Across Industries
Manufacturing and Industrial Automation
In manufacturing, autonomous AI agents are revolutionizing production processes through:
- Predictive maintenance systems that anticipate equipment failures
- Quality control systems that identify defects in real-time
- Production scheduling optimization
- Supply chain management and logistics
- Worker safety monitoring and enhancement
Healthcare and Medical Services
The healthcare sector is seeing significant benefits from autonomous AI agents in:
- Diagnostic assistance and medical imaging analysis
- Patient monitoring and alert systems
- Treatment planning and optimization
- Drug discovery and development
- Healthcare resource allocation and scheduling
Financial Services
In the financial sector, autonomous agents are transforming:
- Trading and investment strategies
- Risk assessment and management
- Fraud detection and prevention
- Customer service and support
- Regulatory compliance monitoring
Transportation and Logistics
The transportation industry is being revolutionized through:
- Autonomous vehicle navigation and control
- Traffic flow optimization
- Fleet management and routing
- Maintenance scheduling and management
- Safety monitoring and enforcement
Key Challenges in Autonomous AI Agents
The development and deployment of autonomous AI agents present numerous complex challenges that span technical, operational, and implementation domains. Understanding these challenges is crucial for anyone working with or developing AI agent systems. Let's explore each major challenge area in detail.
Technical Challenges
Complexity Management
One of the most significant technical challenges lies in managing the inherent complexity of autonomous systems. As AI agents become more sophisticated, their internal systems grow increasingly complex, leading to several critical issues:
The interaction between different components can create unexpected behaviors, much like how a small change in one part of an ecosystem can have far-reaching effects throughout the entire system. For example, a modification in how an agent processes memory might unexpectedly affect its decision-making capabilities in subtle ways that only become apparent under specific conditions.
Debugging these complex systems presents unique challenges because traditional debugging approaches often fall short when dealing with emergent behaviors. It's similar to trying to understand why a city's traffic patterns change - while individual elements might be working correctly, their interactions create complex patterns that are difficult to predict or analyze.
Performance Optimization
Performance optimization in autonomous AI agents involves balancing multiple competing factors:
Resource utilization must be carefully managed across all components. Think of it like managing a company's resources - you need to ensure each department has what it needs while maintaining overall efficiency. An agent might need to decide whether to allocate more resources to processing current information or to planning future actions.
Real-time processing requirements often conflict with the need for thorough analysis. For instance, an autonomous vehicle must balance the need for quick decision-making in traffic with the requirement for careful analysis of potential hazards. This creates a constant tension between speed and accuracy.
Implementation Challenges
System Integration
Integrating autonomous AI agents into existing systems and workflows presents several unique challenges:
Legacy system compatibility often becomes a major hurdle. Imagine trying to add modern smart home features to a house built in the 1950s - while possible, it requires careful planning and often creative solutions to make old and new systems work together harmoniously.
API and protocol standardization becomes crucial when agents need to interact with multiple systems. This is similar to ensuring that people from different countries can communicate effectively - there needs to be a common language or protocol that all systems can understand and use.
Scalability Concerns
As autonomous AI agents are deployed at scale, new challenges emerge:
Resource requirements can grow exponentially with system complexity. Consider how a small dinner party is relatively easy to manage, but organizing a banquet for thousands requires entirely different approaches and resources. Similarly, scaling up AI agents often requires more than just more powerful hardware - it needs fundamental changes in how the system operates.
Load balancing and distribution become critical factors. Just as a growing city needs to carefully plan its infrastructure to handle increased traffic and utility usage, scaled AI systems need sophisticated approaches to handle increased computational loads and data processing requirements.
Operational Challenges
Reliability and Consistency
Maintaining reliable and consistent performance presents ongoing challenges:
Environmental variability can significantly impact agent performance. Much like how weather conditions affect a pilot's ability to fly, changing conditions in an agent's operational environment can affect its ability to function optimally. These changes might be in data quality, user behavior patterns, or system loads.
Ensuring consistent decision-making across different scenarios becomes increasingly difficult as the number of possible situations grows. This is similar to ensuring that a large organization maintains consistent policies across all its branches while still allowing for necessary local adaptations.
Maintenance and Updates
Keeping autonomous AI agents running effectively over time presents several challenges:
System updates must be managed carefully to avoid disrupting ongoing operations. Think of it like performing maintenance on a busy highway - the work needs to be done, but it must be managed in a way that minimizes disruption to regular traffic flow.
Knowledge persistence across updates requires special attention. When updating an agent's systems, we need to ensure that valuable learned experiences and patterns aren't lost, much like how a company wants to retain institutional knowledge even as it modernizes its processes.
Data Management Challenges
Data Quality and Availability
High-quality data is crucial for autonomous AI agents, but ensuring its availability and quality presents several challenges:
Data consistency across sources can be difficult to maintain. Like trying to reconcile different eyewitness accounts of an event, AI agents often need to work with data from various sources that might have inconsistencies or contradictions.
Real-time data processing requirements can strain system resources. Similar to how a news organization must balance the need for quick reporting with fact-checking, AI agents must process incoming data quickly while ensuring accuracy and reliability.
Privacy and Security
Managing data while maintaining privacy and security creates additional challenges:
Data access controls must be carefully balanced with operational needs. This is similar to how a hospital must balance patient privacy with the need for healthcare providers to access medical records efficiently.
Secure data transmission becomes increasingly important as agents interact with more systems. Like securing diplomatic communications, ensuring that data remains protected while moving between systems is crucial for maintaining system integrity.
Adaptation and Learning Challenges
Dynamic Environment Handling
Autonomous AI agents must adapt to changing environments, which presents unique challenges:
Environmental changes can invalidate learned patterns. Much like how a person might need to adjust their driving style when moving to a city with different traffic rules, AI agents need to recognize when their learned behaviors are no longer appropriate and adapt accordingly.
Balancing adaptation with stability becomes crucial. Think of it like updating a recipe - while you want to improve it based on feedback, you don't want to lose what made it successful in the first place.
Continuous Learning Management
Managing how agents learn over time presents several challenges:
Learning rate optimization requires careful tuning. Like how students need different amounts of time to master new concepts, AI agents need carefully calibrated learning rates to effectively improve their performance without becoming unstable.
Preventing negative learning patterns is crucial. Similar to how bad habits can be hard to break, AI agents can develop suboptimal patterns that need to be identified and corrected before they become ingrained in the system.
Conclusion: The Evolution and Future of Autonomous AI Agents
The landscape of autonomous AI agents represents one of the most fascinating intersections of computer science, cognitive systems, and practical application. As we've explored throughout this comprehensive analysis, these agents are far more than simple automated systems - they are sophisticated entities built upon four fundamental components that work in concert to create truly intelligent behavior.
The integration of Profile, Memory, Planning, and Action components creates a system that is greater than the sum of its parts. The Profile component provides the foundation of purpose and identity, much like how a person's core values guide their decisions and actions. This works in harmony with the Memory component, which enables agents to learn and adapt from experience, creating a rich tapestry of knowledge that informs future actions. The Planning component then leverages this knowledge to formulate sophisticated strategies, while the Action component brings these plans to life through precise execution.
What makes autonomous AI agents particularly remarkable is their ability to adapt and evolve. Unlike traditional software systems that operate within rigid parameters, these agents can modify their behavior based on experience while maintaining their core objectives. This adaptability is crucial in today's rapidly changing technological landscape, where the ability to learn and adjust is often as important as raw processing power.
The applications of autonomous AI agents span an impressive range of industries and use cases. In manufacturing, they're transforming production processes through intelligent automation and predictive maintenance. In healthcare, they're enhancing diagnostic capabilities and patient care through sophisticated analysis and monitoring. The financial sector is leveraging these agents for everything from risk assessment to fraud detection, while the transportation industry is being revolutionized through autonomous navigation and fleet management.
However, the path forward is not without its challenges. Technical complexity continues to grow as systems become more sophisticated, requiring ever more innovative approaches to management and optimization. Implementation challenges demand careful consideration of integration and scalability issues. Operational concerns around reliability and maintenance require ongoing attention and refinement. Data management presents its own set of challenges, from ensuring quality and availability to maintaining security and privacy. And the fundamental challenges of adaptation and learning require constant balancing of stability and improvement.
Yet these challenges should not be viewed as roadblocks but rather as opportunities for innovation and advancement. Each challenge we overcome in the development and deployment of autonomous AI agents brings us closer to realizing their full potential. The solutions we develop today will form the foundation for even more sophisticated systems tomorrow.
Looking ahead, the continued evolution of autonomous AI agents promises to further transform how we approach complex problems and tasks. As our understanding of these systems deepens and our technical capabilities advance, we can expect to see even more innovative applications and solutions emerge. The key to success lies in maintaining a balanced approach - one that pushes the boundaries of what's possible while carefully addressing the challenges and limitations we encounter.
Ultimately, autonomous AI agents represent more than just technological advancement; they embody our growing understanding of how to create systems that can learn, adapt, and operate independently while remaining aligned with human needs and objectives. As we continue to refine and improve these systems, their impact on industry, society, and human capability will only grow more profound.
The journey of autonomous AI agents is still in its early stages, and each development brings new insights and possibilities. By understanding their core components, applications, and challenges, we can better appreciate both their current capabilities and their future potential. As we move forward, the continued evolution of these systems will undoubtedly reveal new opportunities and challenges, making this an exciting and dynamic field for years to come.