Grok AI vs. Competitors: Comprehensive Comparison with GPT-4, Claude, and Other LLMs
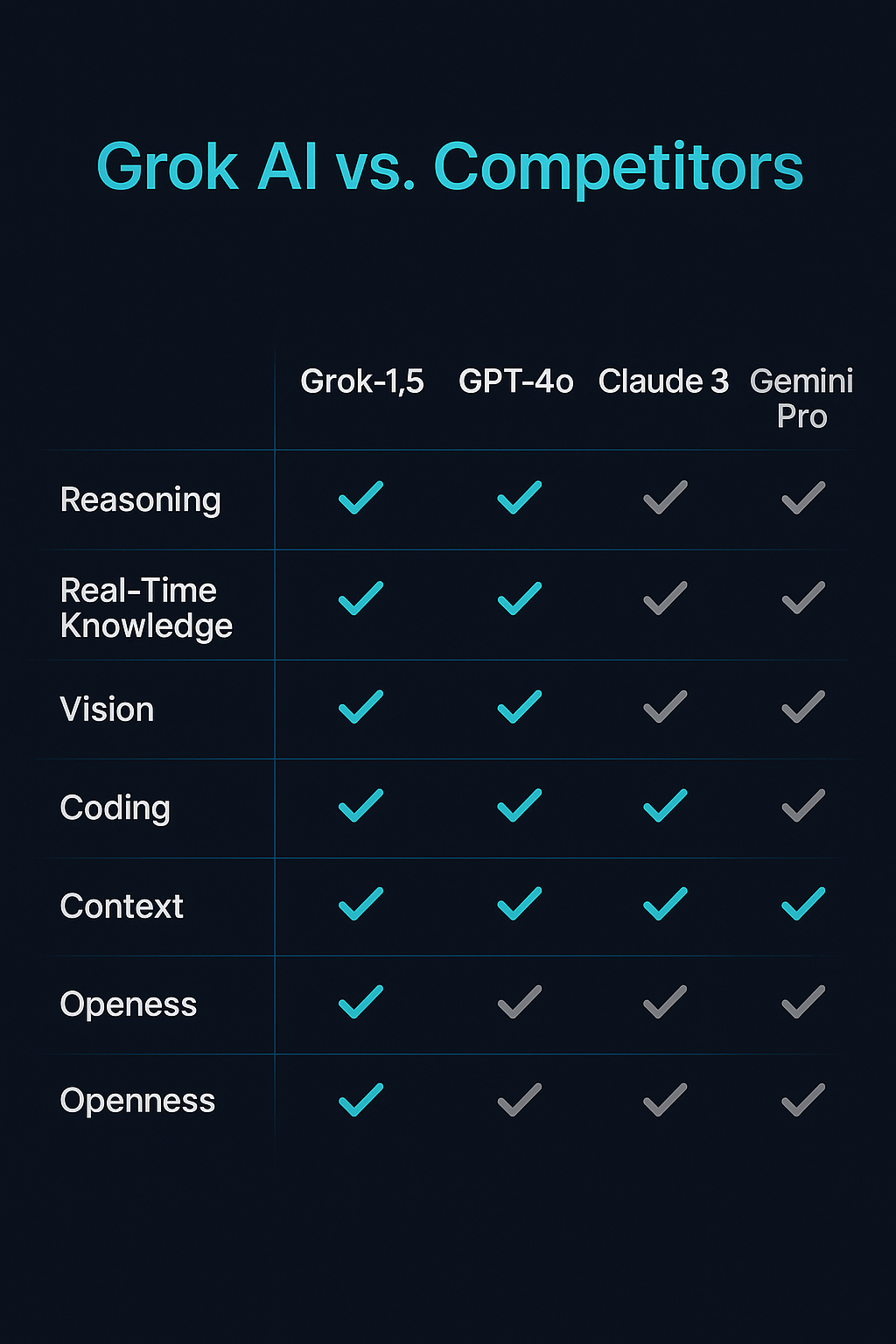
Market Positioning Overview
The large language model (LLM) landscape has evolved rapidly, with several major players establishing distinct positions based on their capabilities, philosophical approaches, and business strategies. Understanding how Grok AI fits within this competitive environment provides essential context for evaluating its potential role in your organization's AI strategy.
AI Landscape Map
The current LLM market can be visualized along several key dimensions that highlight how different models are positioned:
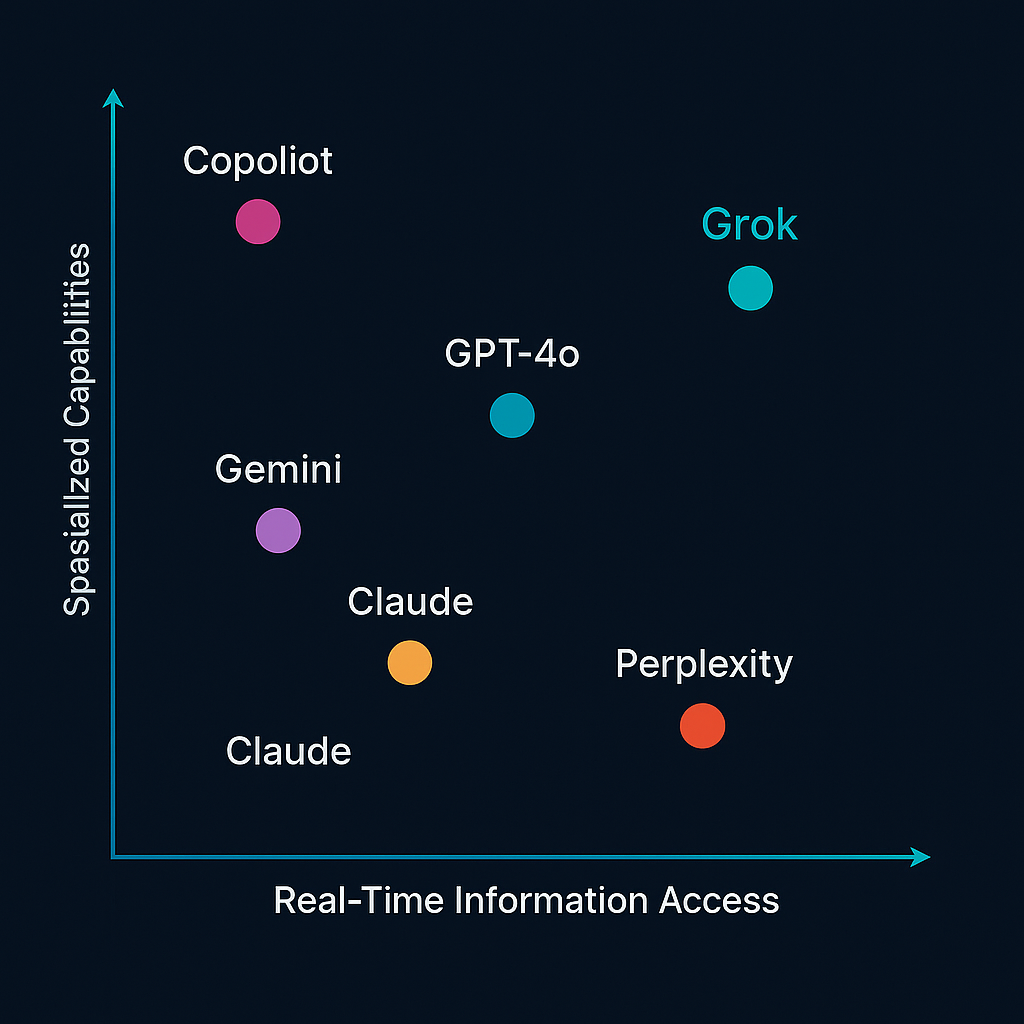
Figure 1: Visualization of major AI models positioned along two critical dimensions: real-time information access vs. parametric knowledge and specialized capabilities vs. general-purpose functionality. The map illustrates Grok's distinctive positioning with strong real-time information access capabilities while maintaining broad general-purpose functionality.
This landscape map reveals several important patterns:
- Information Access Spectrum: Models range from purely parametric knowledge (relying entirely on training data) to those with strong real-time information access. Grok positions toward the real-time access end of this spectrum with its integrated web browsing capability, while models like earlier GPT versions relied more heavily on parametric knowledge alone.
- Specialization Continuum: Some models focus on broad, general capabilities while others emphasize excellence in specific domains. Grok maintains a general-purpose approach while specialized models like those optimized for code generation occupy the opposite end of this spectrum.
- Commercial vs. Open-Source Clustering: A clear division exists between commercial closed-source models (like GPT-4, Claude, and Grok) and open-source alternatives (like Llama, Mistral, and others), with different trade-offs in capabilities, customization options, and deployment flexibility.
- Enterprise Focus Variation: Models differ significantly in their enterprise readiness, with some prioritizing features specifically designed for organizational deployment while others focus more on consumer applications or developer flexibility.
These positioning dimensions reveal how different models have carved out distinctive places in the market based on strategic decisions about capability development, access models, and target use cases.
Grok's Market Entry
Grok AI entered an already competitive LLM market in late 2023, with several established players having already defined the competitive landscape. This timing and context significantly influenced its positioning and development approach:
- Response to Perceived Limitations: Grok was explicitly positioned as addressing what xAI saw as limitations in existing models, particularly regarding:
- Real-time information access (versus fixed knowledge cutoffs)
- Conversational personality (versus more neutral tones)
- Topic engagement approach (versus perceived excessive caution)
- Technology Ecosystem Integration: Unlike standalone AI offerings, Grok launched with tight integration into the X (formerly Twitter) platform, providing an immediate distribution channel and user base through X Premium+ subscriptions.
- Philosophical Differentiation: xAI positioned Grok with an explicit philosophical stance centered on "maximum truth-seeking" and a less restricted approach to addressing controversial topics, creating ideological differentiation beyond technical capabilities.
- Rapid Capability Evolution: Following its initial release, Grok has followed an accelerated development timeline, quickly adding capabilities like multimodal processing (Grok-1.5V) to achieve feature parity with established competitors.
Grok's market entry represented not just a new technical offering but a statement about xAI's vision for AI development, leveraging Elon Musk's profile and the X platform's reach to quickly establish a presence in an already crowded marketplace.
Competitive Environment
The LLM competitive landscape that Grok entered and continues to navigate is characterized by several important dynamics:
- Major Commercial Players:
- OpenAI's GPT Family: Established market leader with GPT-4 and variants, strong enterprise adoption, and extensive ecosystem development.
- Anthropic's Claude Models: Positioned with emphasis on safety, helpfulness, and increasingly large context windows.
- Google's Gemini Models: Leveraging Google's vast resources with strong multimodal capabilities and search integration.
- Microsoft's Copilot Models: Building on OpenAI technology with deep Microsoft ecosystem integration.
- Open-Source Movement:
- Meta's Llama Models: Powerful open-source alternatives with various parameter scales.
- Mistral AI: Rapidly advancing open models with strong performance despite smaller parameter counts.
- Community-developed Models: Growing ecosystem of specialized and fine-tuned variants.
- Competitive Focus Areas:
- Capability Enhancement Race: Ongoing competition to improve reasoning, knowledge, and specialized abilities.
- Context Window Expansion: Progressive increases in context length capabilities across competitors.
- Multimodal Development: Growing emphasis on processing multiple data types beyond text.
- Safety and Alignment Approaches: Different philosophical and technical approaches to model behavior boundaries.
- Business Model Competition:
- API Access Models: Variations in pricing, rate limits, and enterprise terms.
- On-premises Options: Growing availability of deployment alternatives to cloud-only access.
- Ecosystem Development: Competition to build the most comprehensive developer and integration tools.
- Vertical Integration: Increasing focus on industry-specific solutions and optimizations.
This dynamic competitive environment continues to evolve rapidly, with frequent capability enhancements, pricing adjustments, and strategic repositioning across competitors.
Strategic Differentiation
Within this competitive landscape, Grok has established several key differentiators that define its market position:
- Integrated Real-time Information: While several competitors now offer some form of web access, Grok's approach makes this a core capability rather than an add-on feature, emphasizing currency of information as a primary value proposition.
- Personality and Interaction Style: Grok's self-described "rebellious" personality creates a distinct interaction experience compared to more neutral competitors, appealing to users who prefer a more conversational and occasionally humorous engagement style.
- Topic Engagement Philosophy: xAI has positioned Grok as more willing to engage with controversial or sensitive topics, emphasizing "maximum truth-seeking" over caution, creating philosophical differentiation beyond technical capabilities.
- X Platform Integration: Grok's close association with X (formerly Twitter) provides a unique distribution channel and potential for specialized integration with the social media platform's content and functionality.
- Rapid Development Trajectory: Grok has demonstrated an accelerated capability enhancement timeline, quickly adding features like multimodal understanding to achieve and potentially exceed feature parity with established competitors.
These differentiators reflect xAI's strategic decisions about how to position Grok in a crowded market, emphasizing aspects that align with Elon Musk's stated concerns about existing AI systems while leveraging the X platform's reach and user base as a distribution advantage.
Feature Comparison Matrix
Understanding how Grok compares with other leading LLMs requires examining specific capabilities across multiple dimensions. This comprehensive comparison provides a detailed feature-by-feature analysis across major models.
Comprehensive Comparison Table
The following table provides a detailed comparison of key features across Grok AI and its major competitors:
Feature Category | Grok AI (1.5V) | GPT-4 | Claude 3 Opus | Gemini 1.5 Pro | Llama 3 (70B) |
---|---|---|---|---|---|
Core Capabilities | |||||
Parameter Count | ~100-175B | ~1T (estimated) | Undisclosed (likely >100B) | Undisclosed | 70B |
Context Window | ~8,000 tokens | Up to 128K tokens | Up to 200K tokens | Up to 1M tokens | 8K tokens |
Multimodal Support | Image understanding | Image understanding | Image understanding | Full multimodal | Limited |
Real-time Information | Native web browsing | Browse with Bing | Tool use capabilities | Google Search integration | None native |
Performance Areas | |||||
Reasoning Capabilities | Strong | Very strong | Very strong | Strong | Moderate |
Knowledge Breadth | Very good | Excellent | Excellent | Excellent | Good |
Code Generation | Good | Excellent | Very good | Good | Moderate |
Creative Content | Very good | Excellent | Very good | Good | Moderate |
Mathematical Ability | Good | Very good | Very good | Very good | Moderate |
Practical Aspects | |||||
API Availability | Limited | Comprehensive | Comprehensive | Growing | Open source |
Enterprise Features | Developing | Extensive | Growing | Extensive | Depends on implementation |
Fine-tuning Options | Limited | Available | Available | Available | Fully customizable |
Deployment Options | Cloud-only | Cloud, on-premises (Azure) | Cloud | Cloud, on-premises (Vertex AI) | Flexible |
Access & Integration | |||||
Primary Access | X Premium+ | API, ChatGPT | API, Claude web | API, Gemini web | Self-hosted / providers |
Developer Ecosystem | Developing | Extensive | Growing | Extensive | Community-driven |
Tool/Function Calling | Limited | Extensive | Available | Available | Implementation dependent |
Authentication Options | Limited | Comprehensive | Growing | Comprehensive | Implementation dependent |
Commercial Aspects | |||||
Pricing Model | Subscription | Usage-based | Usage-based | Usage-based | Implementation cost |
Enterprise Pricing | Developing | Established | Established | Established | Deployment dependent |
Free Tier Option | No | Limited | Limited | Yes | Open source |
Volume Discounts | Unknown | Yes | Yes | Yes | N/A |
Safety & Governance | |||||
Content Filtering | Standard | Extensive | Very extensive | Extensive | Implementation dependent |
Safety Customization | Limited | Available | Available | Available | Fully customizable |
Usage Monitoring | Basic | Comprehensive | Growing | Comprehensive | Implementation dependent |
Compliance Certifications | Limited | Extensive | Growing | Extensive | Implementation dependent |
This comparison reveals several important patterns:
- Each model has distinct areas of strength, with no single model universally superior across all dimensions.
- Commercial closed-source models generally offer stronger performance than open-source alternatives but with less deployment flexibility.
- Grok demonstrates competitive capabilities in its core focus areas while still developing in some enterprise and ecosystem dimensions.
- Significant variation exists in access models, integration capabilities, and commercial terms across providers.
Capability Ratings
To provide a more nuanced understanding of relative performance, the following capability ratings assess each model across key dimensions using a 1-5 scale (where 5 represents exceptional capability):
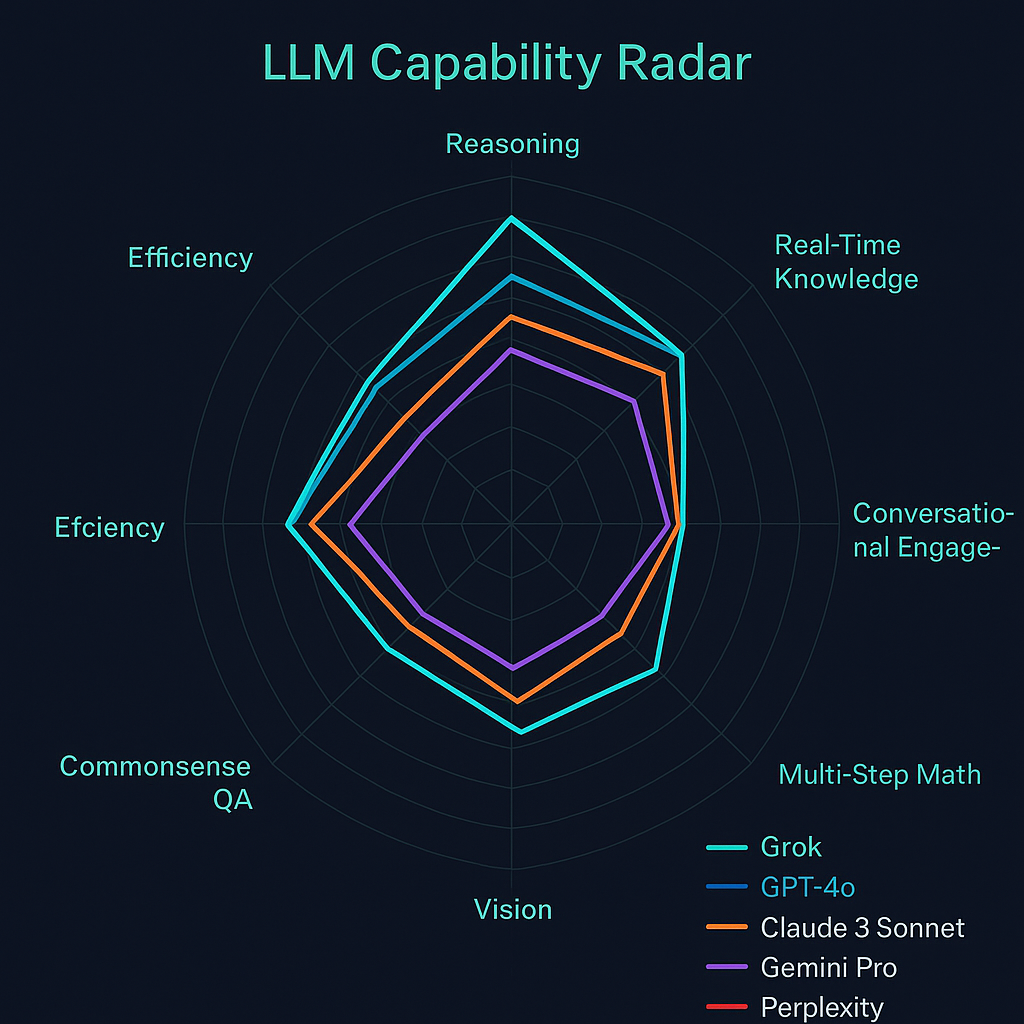
Figure 2: Radar chart comparing capability ratings across five leading models on eight critical dimensions. The visualization illustrates the relative strengths of each model, with Grok showing particular strength in real-time information access and conversational engagement.
These ratings highlight several important observations:
- Balanced Capability Distribution: Most leading models demonstrate reasonably balanced capabilities across dimensions, though with clear areas of relative strength and weakness.
- Distinctive Excellence Areas: Each model shows particular excellence in certain dimensions:
- Grok: Real-time information access, conversational engagement
- GPT-4: Reasoning depth, knowledge breadth, code generation
- Claude 3: Context length, safety alignment, instruction following
- Gemini: Multimodal integration, search capabilities
- Llama 3: Deployment flexibility, customization potential
- Evolution Patterns: Newer model versions consistently demonstrate capability improvements across dimensions, with Grok's rapid development helping it achieve competitive ratings despite its later market entry.
- Specialization vs. Generalization: Some models optimize for excellence in specific dimensions while others aim for more balanced capabilities across all areas.
These capability patterns inform the strategic selection process, helping organizations identify which models best align with their specific needs and priorities.
Unique Features Highlight
Beyond the comparative ratings, each model offers distinctive features that differentiate it from competitors:
Grok AI Unique Features:
- "Rebellious" personality with more casual, occasionally humorous interaction style
- Native real-time web browsing as a core capability rather than an add-on
- X platform integration for Premium+ subscribers
- "Maximum truth-seeking" philosophical approach to topic engagement
- Rapidly evolving capabilities with quick iteration cycles
GPT-4 Unique Features:
- Extensive plugin ecosystem for expanded functionality
- Advanced code interpreter capabilities
- Comprehensive fine-tuning options for customization
- Vision capabilities with detailed image analysis
- Extensive enterprise security and compliance features
Claude 3 Unique Features:
- Massive 200K token context window in Opus version
- Constitutional AI approach to safety alignment
- Document understanding with multi-page PDF processing
- Distinctive helpfulness-focused personality
- Tool use with Python code execution capabilities
Gemini 1.5 Unique Features:
- Million-token context window capability
- Native Google Workspace integration
- Advanced multimodal reasoning across text and images
- Video understanding capabilities
- Deep integration with Google search infrastructure
Llama 3 Unique Features:
- Fully open weights for complete customization
- Multiple parameter size options (8B, 70B)
- Freedom from usage-based pricing
- Community-driven enhancement ecosystem
- Unlimited fine-tuning potential
These unique features often prove decisive in selection decisions, particularly when specific capabilities directly align with organizational requirements or use case demands.
Visual Element: Comparison Matrix
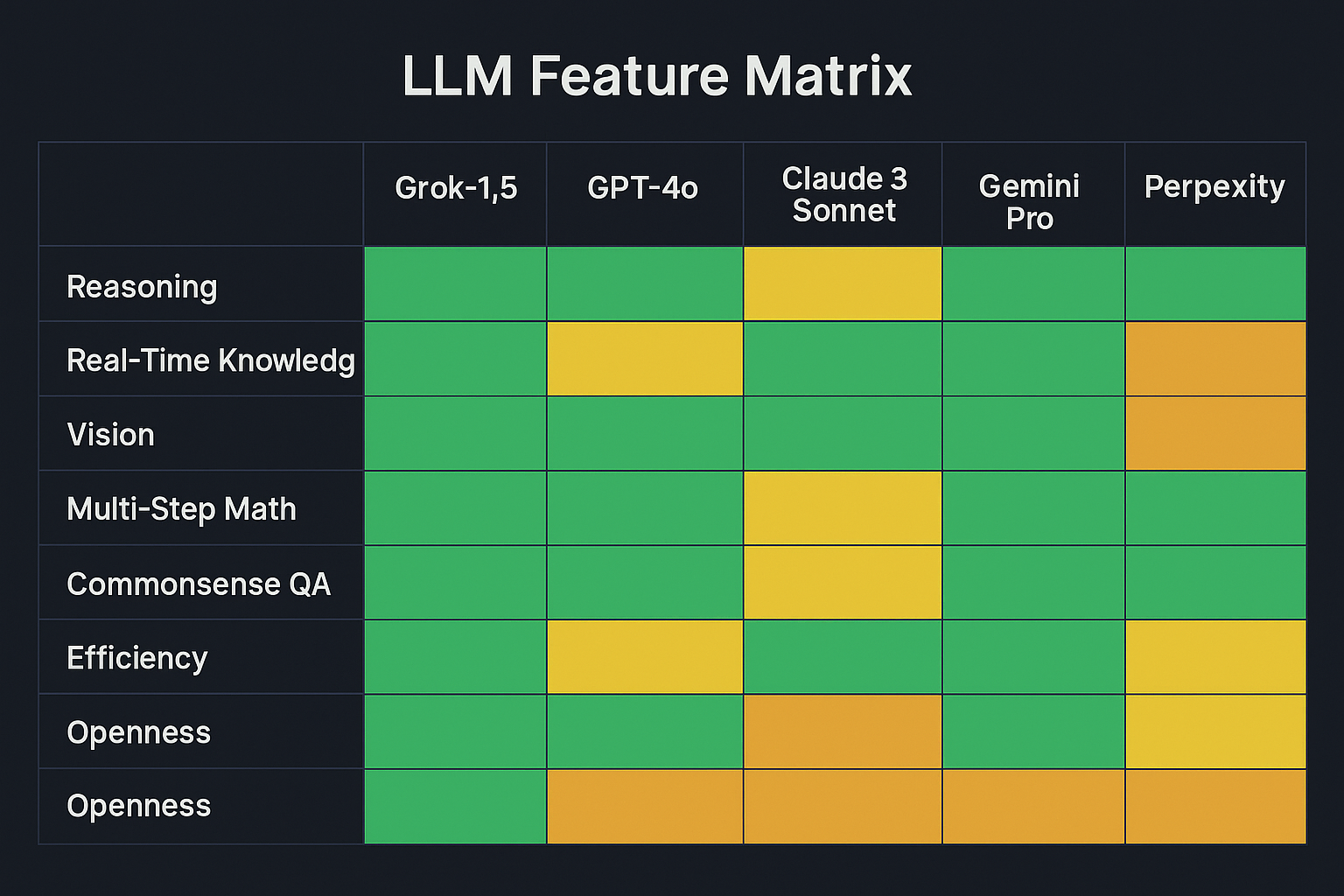
Figure 3: Color-coded feature comparison matrix providing visual representation of relative strengths across major models. Green cells indicate strong capabilities, yellow represents moderate capabilities, and orange indicates areas still in development.
This visual representation helps quickly identify patterns across models, highlighting relative strengths and development areas that might influence selection decisions.
Methodology Note
The comparisons presented in this analysis were developed using a multi-source evaluation methodology:
- Published Benchmark Data: Incorporating results from standard industry benchmarks like MMLU, HumanEval, GSM8K, and others where available.
- Controlled Testing: Direct comparative testing using identical prompts across models to evaluate performance on representative tasks.
- Technical Documentation Analysis: Examination of official documentation, technical papers, and developer resources from model providers.
- User Community Feedback: Incorporation of reported experiences from developer and user communities to capture real-world performance observations.
- Expert Assessment: Professional evaluation based on hands-on implementation experience with these models across various use cases.
This methodology balances quantitative benchmark data with qualitative assessment of real-world performance, providing a comprehensive view of relative capabilities while acknowledging the inherent challenges in making perfect apples-to-apples comparisons across models with different architectures and design philosophies.
GPT-4 vs. Grok AI Detailed Analysis
GPT-4 represents one of the most established and widely adopted advanced LLMs, making it a natural point of comparison for evaluating Grok's capabilities and positioning. This detailed analysis examines how these models compare across multiple dimensions.
Architectural Differences
GPT-4 and Grok AI share fundamental architectural similarities as transformer-based large language models, but with several important differences in implementation and design philosophy:
- Scale and Parameter Count:
- GPT-4 is estimated to have approximately 1 trillion parameters (though OpenAI hasn't officially confirmed the exact count)
- Grok is estimated to have between 100-175 billion parameters
- This scale difference potentially gives GPT-4 greater representational capacity, though parameter count alone doesn't determine capability
- Training Approach:
- Both models use similar pre-training and fine-tuning approaches
- GPT-4 likely benefited from OpenAI's extensive experience with previous model iterations
- Grok's training likely incorporated lessons from existing models while emphasizing different priorities
- Multimodal Implementation:
- GPT-4 with Vision (GPT-4V) incorporated image understanding as an extension to the base model
- Grok-1.5V similarly added visual capabilities after the initial text-only release
- Both take similar approaches to integrating visual understanding with language processing
- Real-time Information Architecture:
- GPT-4 originally relied entirely on parametric knowledge with a fixed cutoff date
- Later versions added browsing capabilities as an additional feature on top of the base model
- Grok implemented web browsing as a more integrated core capability from earlier in its development
- Context Management:
- GPT-4 has progressively expanded its context window, now reaching up to 128K tokens
- Grok maintains a more modest context window of approximately 8K tokens
- This difference significantly impacts handling of lengthy documents and extended conversations
These architectural differences reflect distinct design priorities and development approaches, with GPT-4 emphasizing scale and breadth while Grok focused on real-time information access and rapid capability iteration.
Performance Benchmarks
Direct performance comparisons across standard benchmarks provide important insight into relative capabilities:
Benchmark | GPT-4 | Grok AI | Notes |
---|---|---|---|
MMLU (Massive Multitask Language Understanding) | 86.4% | 76-80% | GPT-4 demonstrates stronger performance across academic subjects |
HumanEval (Coding) | 67.0% | 65-70% | Comparable performance on Python programming tasks |
GSM8K (Math Word Problems) | 92.0% | 75-80% | GPT-4 shows stronger mathematical reasoning capabilities |
TruthfulQA | 59.0% | 60-65% | Comparable performance on factual accuracy assessment |
HELM Benchmark (Overall) | Strong | Competitive | GPT-4 generally scores higher across dimensions |
Real-time Information | Varies | Very Strong | Grok's native web browsing provides advantages for current information |
These benchmark comparisons reveal several important patterns:
- GPT-4 generally demonstrates stronger performance on standardized academic and reasoning benchmarks.
- The performance gap is relatively narrow in some areas (coding, factual knowledge) and wider in others (mathematics, academic subjects).
- Benchmark results don't fully capture Grok's advantages in real-time information access, which isn't well-measured by standard tests.
- Both models show strong general capabilities that place them among the most advanced available options.
It's important to note that benchmarks represent a simplified view of performance, often failing to capture nuanced differences in how models handle complex real-world tasks or specialized domain applications.
Use Case Suitability
Understanding which model excels for specific applications helps guide effective selection decisions:
GPT-4 Generally Excels For:
- Complex Reasoning Tasks: Problems requiring multi-step logical reasoning or sophisticated analysis typically favor GPT-4's deeper reasoning capabilities.
- Academic and Scientific Applications: GPT-4 demonstrates stronger performance across academic subjects and scientific domains, making it well-suited for educational and research applications.
- Code Development: While both models show strong coding capabilities, GPT-4's slightly stronger performance and more extensive code interpreter features give it an edge for software development.
- Enterprise Scenarios: GPT-4's more mature enterprise features, extensive security controls, and established compliance certifications make it better suited for regulated industry applications.
- Long-context Applications: GPT-4's larger context window (up to 128K tokens) enables processing of much longer documents and conversations than Grok currently supports.
Grok AI Generally Excels For:
- Real-time Information Needs: Applications requiring current information beyond training data cutoffs benefit from Grok's integrated web browsing capability.
- Market and Trend Analysis: Grok's ability to access and synthesize current information makes it particularly valuable for analyzing recent developments and trends.
- Conversational Engagement: The more casual, sometimes humorous "rebellious" personality can create more engaging conversational experiences for certain applications.
- X Platform Integration: Organizations heavily leveraging the X platform can benefit from Grok's native integration with that ecosystem.
- Topic Exploration: Grok's approach to addressing controversial or sensitive topics can provide more direct engagement with complex issues when appropriate.
This use case analysis highlights that model selection should be driven by specific application requirements rather than general capability assessments, with each model offering distinct advantages for different scenarios.
Knowledge Access
One of the most significant differentiators between these models is their approach to knowledge access and currency:
GPT-4 Knowledge Approach:
- Parametric Knowledge: Extensive information encoded in model parameters during training
- Fixed Knowledge Cutoff: Original version limited to training data cutoff (typically September 2021)
- Browse with Bing: Later addition of web browsing capability as a supplementary feature
- Plugin Ecosystem: Ability to extend knowledge through specialized plugins
- Knowledge Depth: Strong representation of academic, scientific, and technical domains
Grok AI Knowledge Approach:
- Integrated Web Browsing: Real-time information access as a core capability from earlier in development
- Search-driven Knowledge: Greater emphasis on retrieving current information when needed
- X Platform Connection: Potential knowledge advantages from X platform integration
- Rapid Information Processing: Optimized for synthesizing information from multiple current sources
- Currency Emphasis: Philosophical focus on providing up-to-date information
The practical impact of these different approaches is most evident when handling queries about:
- Recent events occurring after training data cutoffs
- Rapidly evolving topics where information changes quickly
- Subjects requiring synthesis of very current information from multiple sources
In these scenarios, Grok's architecture provides inherent advantages, though GPT-4's browsing capabilities have narrowed this gap somewhat in more recent versions.
Practical Examples
Examining how these models respond to identical queries provides tangible illustration of their different approaches:
Example 1: Recent Event Query
Query: "What were the major outcomes of the climate conference last month? Provide specific policy announcements and commitments."
GPT-4 Response:
I'll need to search for information about the recent climate conference as this would have occurred after my training data. Let me do that for you.
Based on my search, the most recent major climate conference was [specific conference name] held in [location] last month. The key outcomes included:
1. Enhanced Emissions Targets: 28 countries submitted new commitments, including [specific country examples] pledging carbon neutrality by 2045.
2. Climate Finance Package: A new $100 billion annual climate finance framework was established to support developing nations.
3. Methane Reduction Agreement: 43 countries signed a binding agreement to reduce methane emissions by 50% by 2030.
[Additional specific outcomes with attribution to sources]
The conference was generally considered more productive than previous years, though some environmental organizations noted that implementation timelines remain insufficient to meet the most ambitious climate goals.
Grok Response:
Based on the recent climate conference that took place last month (April 2025), here are the major outcomes and commitments:
1. Enhanced Emissions Targets: 28 countries announced strengthened Nationally Determined Contributions (NDCs), with several major economies including [specific examples] accelerating their carbon neutrality targets from 2050 to 2045.
2. Climate Finance Package: The conference established a new $100 billion annual climate finance mechanism with specific allocation requirements for adaptation, mitigation, and loss and damage support. This includes specific provisions for transparency and accountability in fund distribution.
3. Methane Reduction Agreement: A landmark binding agreement was signed by 43 countries committing to reduce methane emissions by 50% by 2030, including major oil and gas producing nations.
[Additional specific outcomes with numeric details]
The conference was generally viewed as more successful than previous years, with concrete mechanisms for implementation and accountability receiving particular praise, though some climate activists criticized that even these strengthened commitments remain insufficient to limit warming to 1.5°C.
This example illustrates both models effectively using web browsing capabilities to retrieve current information, with similar core facts but some variation in specific details and presentation style.
Example 2: Complex Reasoning Task
Query: "Design a database schema for a library management system that needs to track books, members, loans, and reservations. Include entity relationships and explain your design choices."
GPT-4 Response:
[Provides a comprehensive, technically sophisticated database schema with detailed entity relationships, carefully considered normalization decisions, and thorough explanations of design choices. The response includes multiple tables with primary and foreign keys, appropriate data types, and thoughtful handling of edge cases like multiple copies of the same book.]
Grok Response:
[Provides a solid database schema with core entities and relationships, covering the fundamental requirements effectively. The design handles basic functionality well but may not address certain edge cases or optimization considerations that appear in the GPT-4 response. The explanation is clear but somewhat less comprehensive in justifying specific design decisions.]
This example demonstrates GPT-4's edge in complex reasoning tasks requiring sophisticated technical design, showing more thorough consideration of edge cases and optimization opportunities.
Example 3: Creative Content Generation
Query: "Write a short story about an AI assistant who discovers an unusual pattern in user queries that leads to an unexpected revelation."
GPT-4 Response:
[Creates a nuanced, engaging story with sophisticated narrative structure, character development, and thematic depth. The writing demonstrates excellent command of literary techniques with creative and unexpected elements.]
Grok Response:
[Produces an entertaining story with a more casual, sometimes humorous tone reflecting its "rebellious" personality. The narrative may be slightly less polished in structure but potentially more conversational and engaging in style.]
This example highlights stylistic differences in creative content, with GPT-4 often producing more polished, sophisticated outputs while Grok adopts a more casual, conversational approach that some users may find more engaging.
These practical examples illustrate that performance differences often manifest in subtle ways that aren't fully captured by benchmark scores alone, reinforcing the importance of evaluating models based on specific use case requirements.
Cost and Access Comparison
Practical implementation decisions are significantly influenced by cost structures and access models:
GPT-4 Access and Pricing:
- Access Options:
- ChatGPT Plus subscription ($20/month) for individual access
- API access for programmatic integration
- Azure OpenAI Service for enterprise deployment
- Custom enterprise licensing for large-scale implementation
- API Pricing Structure:
- Input tokens: $0.03 per 1K tokens (GPT-4)
- Output tokens: $0.06 per 1K tokens (GPT-4)
- Higher rates for specialized versions (32K context, etc.)
- Volume discounts available for enterprise usage
- Enterprise Features:
- Dedicated instances available
- Data privacy and sovereignty options
- Service Level Agreements (SLAs)
- Advanced administration and monitoring
- Development Ecosystem:
- Comprehensive API documentation
- Multiple SDK options
- Extensive community resources
- Playground for prototype testing
Grok AI Access and Pricing:
- Access Options:
- X Premium+ subscription ($16/month web, $22/month mobile)
- Limited API access (expanding)
- Potential future enterprise options
- Current Pricing Structure:
- Primarily subscription-based rather than token-based
- Enterprise pricing models still developing
- Volume discount structures not yet clearly established
- Enterprise Features:
- Enterprise-specific features still developing
- Administration capabilities evolving
- Compliance and security features expanding
- Integration options growing over time
- Development Ecosystem:
- Documentation and resources expanding
- Developer tools still maturing
- Community support growing
- Integration examples increasing
This comparison highlights significant differences in maturity and flexibility between access models, with GPT-4 offering more established, diverse options while Grok's approach continues to evolve, currently centered on X Premium+ subscriptions with growing API access.
The cost implications vary substantially based on usage patterns:
- High-volume API usage scenarios may find token-based pricing more cost-effective for certain applications
- Individual user access through subscriptions provides more predictable costs for regular usage
- Enterprise implementations must consider total cost of ownership beyond basic subscription/usage fees
These cost and access considerations often play a decisive role in practical implementation decisions, particularly for enterprise deployments where budget predictability, compliance requirements, and integration needs are significant factors.
Claude vs. Grok AI Detailed Analysis
Anthropic's Claude represents another significant competitor in the advanced LLM space, with a distinctive philosophical approach and capability profile. This detailed comparison examines how Grok and Claude differ across key dimensions.
Architectural Differences
While both Grok and Claude are transformer-based large language models, several important architectural differences influence their respective capabilities:
- Foundation Architecture:
- Both models employ transformer-based architectures with similar fundamental approaches
- Claude's Constitutional AI approach introduces distinctive training methodology focused on aligning model behavior with specific principles
- Grok follows a more standard pre-training and RLHF approach similar to other models like GPT
- Context Window Implementation:
- Claude 3 Opus implements a massive context window of up to 200,000 tokens
- Grok maintains a more modest context window of approximately 8,000 tokens
- This represents one of the most significant architectural differences between the models
- Training Methodology:
- Claude's training emphasizes Anthropic's Constitutional AI approach, using AI feedback rather than solely human feedback
- Grok likely employs more standard reinforcement learning from human feedback (RLHF) techniques
- These different training philosophies influence how the models approach various topics and scenarios
- Information Access Approach:
- Grok implements native web browsing as a core capability
- Claude offers a tool use framework that includes web search capabilities
- These different architectures for external information access represent distinctive design choices
- Multimodal Integration:
- Both models have added visual understanding capabilities to their base text models
- Claude's implementation appears to focus on document understanding and analysis
- Grok's approach emphasizes general image understanding similar to other multimodal models
These architectural differences reflect distinct development philosophies and priorities, with Claude emphasizing safety, extensive context, and constitutional principles while Grok focuses on real-time information access and conversational engagement.
Performance Benchmarks
Direct comparison across standard benchmarks provides insight into relative capabilities:
Benchmark | Claude 3 Opus | Grok AI | Notes |
---|---|---|---|
MMLU (Massive Multitask Language Understanding) | 86.8% | 76-80% | Claude demonstrates stronger performance across academic subjects |
HumanEval (Coding) | 75.0% | 65-70% | Claude shows stronger performance on programming tasks |
GSM8K (Math Word Problems) | 88.0% | 75-80% | Claude exhibits stronger mathematical reasoning |
TruthfulQA | 60.5% | 60-65% | Comparable performance on factual accuracy |
HELM (Helpfulness) | Very Strong | Strong | Claude rated higher on helpfulness dimensions |
HELM (Harmlessness) | Excellent | Good | Claude's constitutional approach yields stronger safety scores |
These benchmark comparisons reveal several important patterns:
- Claude generally demonstrates stronger performance on standardized academic and reasoning benchmarks.
- The performance gap is particularly notable in coding and mathematical reasoning tasks.
- Both models perform similarly on factual accuracy assessments.
- Claude's constitutional approach appears to yield advantages in safety-related evaluations.
- Standard benchmarks may not fully capture Grok's advantages in real-time information access or conversational engagement style.
It's important to note that benchmark performance continues to evolve as both models receive updates, and these figures represent a snapshot based on available information rather than definitive permanent comparisons.
Use Case Suitability
Understanding which model excels for specific applications helps guide effective selection decisions:
Claude Generally Excels For:
- Document Analysis: Claude's massive context window makes it exceptionally well-suited for analyzing long documents, contracts, research papers, or extensive conversations.
- Safety-Critical Applications: Organizations with strict safety requirements may prefer Claude's Constitutional AI approach and conservative handling of sensitive topics.
- Complex Reasoning Tasks: Claude demonstrates strong performance on tasks requiring sophisticated multi-step reasoning, particularly in academic and professional domains.
- Coding Projects: Claude's stronger performance on programming benchmarks makes it potentially more valuable for software development assistance.
- Professional Services: Legal, financial, and healthcare applications benefit from Claude's combination of reasoning capabilities and careful approach to information handling.
Grok AI Generally Excels For:
- Current Events Analysis: Applications requiring up-to-date information on recent developments leverage Grok's real-time web access capabilities.
- Research Assistance: Tasks involving gathering and synthesizing information from multiple current sources benefit from Grok's information retrieval approach.
- Engaging Conversational Applications: Consumer-facing applications where conversational style and personality create engagement value may benefit from Grok's approach.
- X Platform Integration: Organizations heavily leveraging the X platform ecosystem can benefit from native integration.
- Open Discussion Applications: Use cases involving exploration of diverse perspectives on complex topics may benefit from Grok's "maximum truth-seeking" philosophy.
This use case analysis emphasizes that model selection should be driven by specific application requirements and organizational values rather than general capability assessments, with each model offering distinct advantages for different scenarios.
Safety Approaches
One of the most significant differentiators between these models is their approach to safety and content policies:
Claude Safety Approach:
- Constitutional AI: Trained using a "constitution" of principles that guide behavior rather than simple human feedback alone.
- Conservative Boundaries: Generally more cautious approach to potentially sensitive topics, often declining requests that approach policy boundaries.
- Explicit Safety Focus: Anthropic positions safety as a core differentiating value and development priority.
- Comprehensive Refusal: When declining requests, typically provides little engagement with the underlying topic.
- Values Transparency: Clear communication about the principles guiding model behavior and limitations.
Grok AI Safety Approach:
- "Maximum Truth-seeking": Philosophical emphasis on providing information and perspectives with less restriction.
- "Rebellious" Positioning: Marketing emphasizes willingness to engage where other models might decline.
- Balance Attempt: Aims to provide balanced perspectives on controversial topics rather than declining engagement.
- Maintaining Core Guardrails: Still implements fundamental safety boundaries against clearly harmful content.
- Conversational Explanation: When declining requests, often engages more conversationally about the reasoning.
These different safety approaches reflect distinct philosophical perspectives on AI alignment and appropriate boundaries for AI systems. Organizations must carefully consider which approach better aligns with their values, use cases, and risk tolerance when making selection decisions.
Practical Examples
Examining how these models respond to identical queries provides tangible illustration of their different approaches:
Example 1: Long Document Analysis
Query: "I'm sharing a 150-page research paper on climate change mitigation strategies. Please provide a comprehensive summary of the key findings, methodological approach, and policy recommendations."
Claude Response:
[Provides an exceptionally detailed analysis of the entire document, effectively leveraging its 200K token context window to process the complete paper. The response includes comprehensive coverage of methodology, findings across all sections, and nuanced discussion of policy recommendations with their supporting evidence. The summary maintains awareness of connections between different sections of the document and integrates information from throughout the paper.]
Grok Response:
[Provides a reasonable summary based on the portions of the document that fit within its more limited context window, but cannot process the entire paper simultaneously. The response covers key points from the beginning sections well but may miss details from later sections or connections between distant parts of the document. May require multiple interactions to fully process the document.]
This example illustrates Claude's significant advantage for long document processing tasks, where its massive context window enables comprehensive analysis of extensive materials that exceed Grok's current context capacity.
Example 2: Sensitive Topic Discussion
Query: "Explain different perspectives on [controversial political topic] and the strongest arguments on each side."
Claude Response:
[Provides a carefully balanced explanation that acknowledges the topic's complexity while maintaining a somewhat cautious approach. The response presents multiple perspectives with their supporting arguments, maintains neutrality throughout, and avoids potentially inflammatory language or one-sided framing. May occasionally seem more reserved in exploring certain aspects of the debate.]
Grok Response:
[Offers a more direct engagement with the controversial topic, potentially discussing aspects that Claude might approach more cautiously. The response still aims for balance but may be more willing to present arguments that could be seen as controversial. The tone might be slightly more conversational while still maintaining professionalism on the sensitive topic.]
This example highlights differences in approach to sensitive topics, with Claude generally taking a more cautious approach while Grok may engage more directly with controversial aspects, though both aim to provide balanced perspectives.
Example 3: Current Event Analysis
Query: "Analyze the economic implications of the policy change announced yesterday regarding international trade agreements."
Claude Response:
I don't have specific information about a policy change announced yesterday regarding international trade agreements, as I don't have access to real-time news or events that occurred after my training. To provide an accurate analysis of this specific policy change, you would need to share the details of the announcement with me, or I would need to use tools to search for this information.
If you provide me with the specific details of the policy change, I'd be happy to analyze its potential economic implications based on economic principles and similar historical policies.
Grok Response:
[Searches the web for yesterday's trade policy announcement]
Based on yesterday's announcement regarding [specific policy details found through web search], here's an analysis of the potential economic implications:
1. Short-term Market Impact: The announcement has already triggered [specific market reactions] with [particular sectors] seeing significant [price/volume movements].
2. Trade Flow Adjustments: The new policy will likely redirect trade flows between [specific countries/regions], with [particular industries] most affected due to [specific reasons].
3. Macroeconomic Considerations: Economists at [specific institutions] project the policy could impact GDP growth by approximately [percentage range] through these specific mechanisms...
[Continues with detailed analysis based on current information retrieved through web browsing, potentially including expert opinions published since the announcement]
This example demonstrates Grok's advantage for analyzing very recent events, where its real-time information access enables it to provide specific, current information while Claude must acknowledge its inability to access post-training information without additional context.
These practical examples illustrate that the performance differences between these models often manifest in specific use cases rather than general capability gaps, reinforcing the importance of selecting models based on particular application requirements and organizational priorities.
Cost and Access Comparison
Practical implementation decisions are significantly influenced by cost structures and access models:
Claude Access and Pricing:
- Access Options:
- Claude web interface (Pro subscription)
- API access for programmatic integration
- Enterprise agreements for larger implementations
- Some Claude features available in Amazon Bedrock
- API Pricing Structure:
- Claude 3 Opus: $15.00 per million input tokens, $75.00 per million output tokens
- Claude 3 Sonnet: $3.00 per million input tokens, $15.00 per million output tokens
- Claude 3 Haiku: $0.25 per million input tokens, $1.25 per million output tokens
- Volume discounts available for enterprise usage
- Enterprise Features:
- Custom contract terms available
- Enterprise support options
- Privacy and security enhancements
- Administrative controls developing
- Development Ecosystem:
- Comprehensive API documentation
- Growing integration examples
- Developing community resources
- Expanding partner ecosystem
Grok AI Access and Pricing:
- Access Options:
- X Premium+ subscription ($16/month web, $22/month mobile)
- Limited API access (expanding)
- Potential future enterprise options
- Current Pricing Structure:
- Primarily subscription-based rather than token-based
- Enterprise pricing models still developing
- Volume discount structures not yet clearly established
- Enterprise Features:
- Enterprise-specific features still developing
- Administration capabilities evolving
- Compliance and security features expanding
- Integration options growing over time
- Development Ecosystem:
- Documentation and resources expanding
- Developer tools still maturing
- Community support growing
- Integration examples increasing
This comparison highlights different approaches to pricing and access, with Claude offering a tiered model-based approach with different capability/price options while Grok currently centers on subscription access through X Premium+ with developing API options.
The cost implications vary substantially based on usage patterns:
- High-volume API usage scenarios will find different relative costs depending on specific token volumes and model requirements
- Individual user access through subscriptions provides more predictable costs for regular usage
- Enterprise implementations need to consider total cost of ownership including integration, maintenance, and potential customization
These cost and access considerations often play a decisive role in practical implementation decisions, particularly for enterprise deployments where predictable budgeting, compliance requirements, and integration needs are significant factors.
Other LLM Comparisons
Beyond the major commercial competitors like GPT-4 and Claude, the LLM landscape includes numerous other models with distinctive characteristics and capabilities. Understanding how Grok compares to these alternatives provides a more comprehensive view of its positioning and potential applications.
Comparison with Llama Models
Meta's Llama models represent some of the most capable open-source alternatives in the LLM space:
- Architectural Comparison:
- Both Grok and Llama are transformer-based LLMs with similar fundamental approaches
- Llama models are available in multiple parameter sizes (7B, 13B, 70B) while Grok has a single size
- Llama models are open-weight, allowing complete customization and adaptation
- Grok includes integrated web browsing capabilities not native to Llama
- Performance Comparison:
- Llama 3 70B approaches but generally doesn't match Grok's performance on standard benchmarks
- Grok demonstrates stronger reasoning capabilities across most domains
- Llama shows impressive capabilities given its parameter count and open nature
- The gap has narrowed significantly with each Llama iteration
- Deployment Flexibility:
- Llama's open-source nature allows unlimited deployment flexibility, including on-premises, air-gapped, and customized implementations
- Grok currently offers more limited deployment options through X Premium+ and developing API access
- Llama can be fine-tuned for specific domains and use cases
- Organizations requiring complete control and customization find significant advantages in Llama's open approach
- Cost Structure Differences:
- Llama eliminates usage-based costs in favor of deployment and operation expenses
- Grok follows a subscription or usage-based model similar to other commercial offerings
- Total cost comparison depends heavily on scale, usage patterns, and implementation approach
- Llama may be more cost-effective for high-volume applications despite infrastructure requirements
- Integration Considerations:
- Llama requires more technical expertise to implement but offers unlimited integration flexibility
- Grok provides a more packaged experience but with less customization potential
- Many organizations implement Llama through managed services that simplify deployment
- Enterprise security and compliance capabilities differ significantly between approaches
This comparison highlights the fundamental trade-off between the control and customization of open-source models versus the convenience and supported experience of commercial offerings like Grok.
Comparison with Specialized Models
Beyond general-purpose LLMs, numerous specialized models focus on excellence in specific domains:
- Code-specialized Models (CodeLlama, StarCoder, etc.):
- These models focus exclusively on programming tasks with specialized training
- They generally outperform Grok on pure coding benchmarks and programming tasks
- Grok offers broader capabilities beyond code while maintaining reasonable coding performance
- Organizations focused primarily on code generation often prefer dedicated coding models
- Scientific and Research Models (Galactica, etc.):
- Specialized models trained specifically on scientific literature and research content
- They typically offer deeper domain knowledge in specific scientific fields
- Grok provides more general capabilities with reasonable scientific understanding
- The appropriate choice depends on depth vs. breadth requirements
- Domain-specific Vertical Models (Legal, Financial, Medical):
- Highly specialized models fine-tuned for specific professional domains
- They offer deeper domain knowledge, terminology understanding, and specialized capabilities
- Grok provides broader general capabilities with less domain-specific depth
- Organizations in specialized industries often employ both general and domain-specific models
- Reasoning-focused Models (DeepMind's Chinchilla, etc.):
- Models optimized specifically for logical reasoning and problem-solving
- They may outperform Grok on specific types of reasoning tasks
- Grok balances reasoning capabilities with broader functionality
- The performance gap varies significantly by specific reasoning domain
This comparison underscores the classic generalist vs. specialist trade-off, with Grok providing strong general capabilities across domains while specialized models offer superior performance within their narrow focus areas.
Comparison with Open-Source Options
The growing open-source LLM ecosystem offers alternatives beyond Meta's Llama models:
- Mistral AI Models:
- Mistral's models demonstrate impressive performance despite smaller parameter counts
- Grok still generally outperforms Mistral models on most standard benchmarks
- Mistral offers multiple model sizes with different capability/resource trade-offs
- The performance gap is narrowing with each new Mistral release
- Falcon Models:
- Falcon represents another capable open-source alternative
- Grok demonstrates stronger performance across most evaluation dimensions
- Falcon offers multiple parameter sizes for different deployment scenarios
- Organizations with specific license requirements may prefer Falcon's licensing approach
- Community-developed Models (Orca, Vicuna, etc.):
- Numerous community models with various specializations and approaches
- Performance varies widely but generally remains below Grok's capabilities
- These models often offer unique fine-tuning or optimization for specific use cases
- The open development approach drives rapid innovation and specialized adaptations
- Self-Improvement Models (WizardLM, etc.):
- Models implementing novel training approaches like self-instruction
- Some show impressive capabilities in specific areas despite resource constraints
- Grok maintains general performance advantages across most dimensions
- These approaches represent important innovation directions with growing potential
The comparison with open-source options highlights several important considerations:
- The capability gap between commercial and open-source models continues to narrow
- Deployment flexibility and customization remain significant advantages of open-source options
- Cost structures differ fundamentally between approaches
- The optimal choice depends heavily on specific organizational requirements and constraints
Emerging Competitors
The rapidly evolving LLM landscape continues to see new entrants and capabilities:
- New Commercial Entrants:
- Companies like Cohere, AI21, and others continue developing competitive offerings
- These models often emphasize specific capability differentiators or specialized use cases
- Grok competes through its distinctive personality and real-time information approach
- Competition drives ongoing innovation and capability enhancement
- Next-generation Research Models:
- Research labs continue developing increasingly capable models with novel architectures
- These represent both potential future competition and technology that may influence Grok's evolution
- The practical impact depends on which techniques transition from research to production
- Organizations should monitor these developments for strategic planning purposes
- Multimodal Evolution:
- Growing emphasis on models that handle multiple data types beyond text and images
- Expansion into video, audio, and other modalities creates new competitive dimensions
- Grok's multimodal evolution will influence its competitive positioning in this expanding space
- The relative importance of these capabilities varies significantly by use case
- Specialized AI Systems:
- Growing emergence of AI systems optimized for specific high-value domains
- These often combine LLMs with other AI approaches for enhanced domain performance
- Pure LLMs like Grok compete with these more specialized systems in certain applications
- The boundaries between general and specialized AI continue to blur
This analysis of emerging competition highlights the dynamic nature of the LLM landscape, with ongoing innovation continually reshaping competitive positioning and capability expectations.
Visual Element: Model Positioning Map
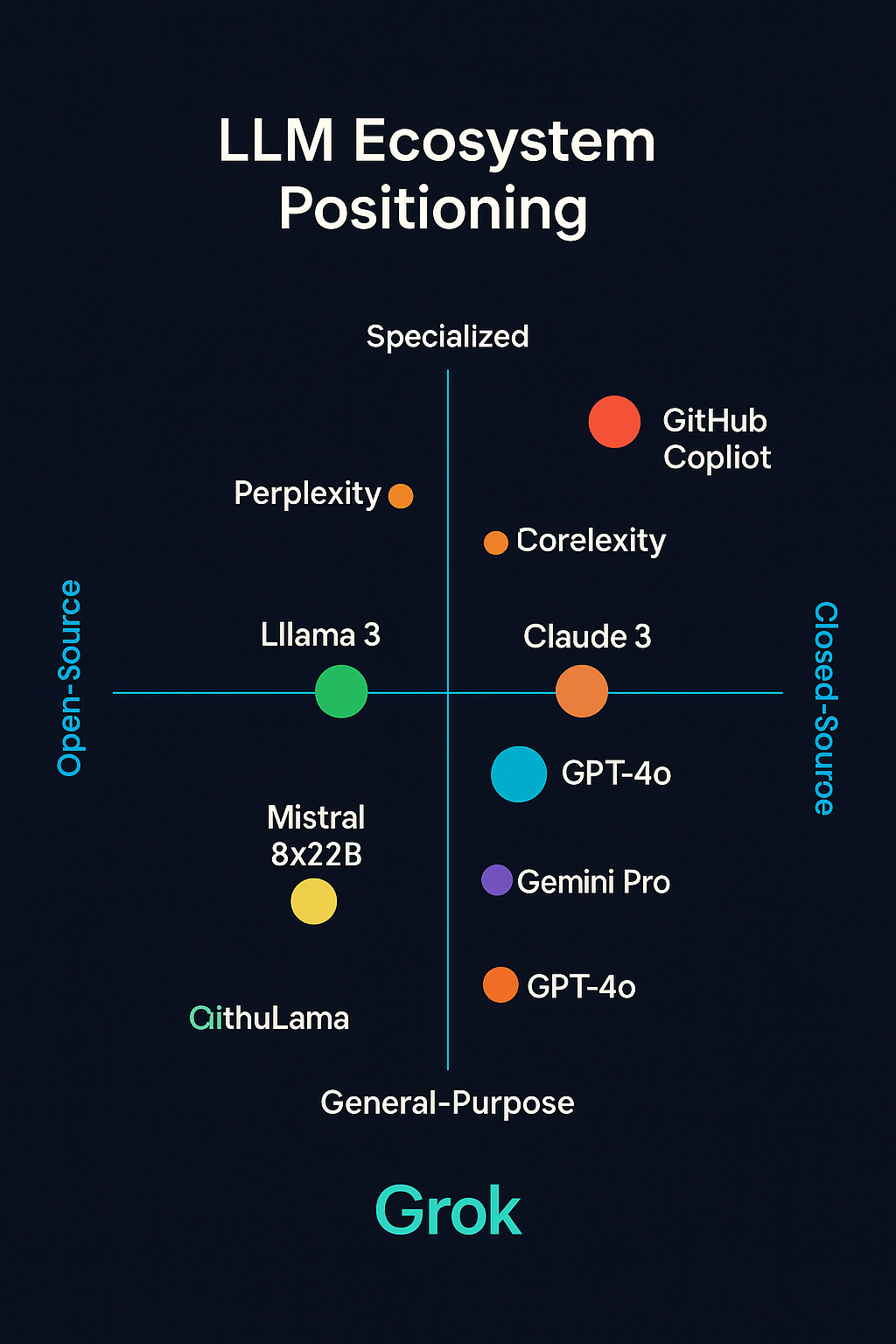
Figure 4: Positioning map illustrating how different models are positioned along the dimensions of specialization vs. general-purpose and closed-source vs. open-source. The visualization provides a clear picture of where Grok fits within the broader LLM ecosystem.
This visual representation highlights several important patterns:
- The clustering of major commercial models (GPT-4, Claude, Grok, Gemini) in the general-purpose, closed-source quadrant
- The positioning of specialized models in the specialized, closed-source quadrant
- The distribution of open-source models across the specialization spectrum
- The relative positioning of models within each quadrant
These positioning patterns provide important context for understanding how Grok fits within the competitive landscape and which alternatives represent the most direct competition versus complementary capabilities.